Hive.Aero develops autonomous drone solutions for various industries, and one of the company's most promising use cases is video analytics for analyzing city traffic and parking spaces. An important requirement for such analytics is the ability to provide real-time processing of video data onboard the drone.
Task:
In the case of Hive.Aero, neural networks were being used to segment the input images and recognize objects in the video. The vehicle detection solution was implemented based on the YOLO_v5s neural network architecture, while the requirement was to achieve maximum acceleration on the Nvidia Jetson TX2 with an accuracy loss of not more than 1%.
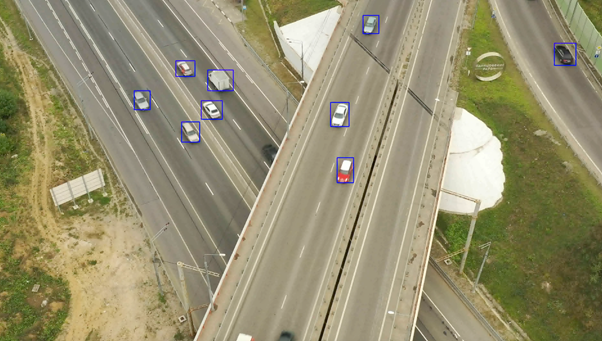
Main technical parameters
● accuracy of object detection and segmentation - mAP;
● the time it takes to process one frame - latency (ms/image).
Input data from the customer
● Hardware - Nvidia Jetson TX2;
● Inference engine - TensorRT;
● The architecture of the neural network - YOLO_V5s
● Processing time per frame - 108 ms/image
Target:
reduce processing time per frame as much as possible, without changing the hardware and with an accuracy loss of less than 1%.
Results:
Taking into account Hive’s requirements, enot.ai accelerated the neural network 2.1 times. After optimization, the time to process a video frame was reduced to 51 milliseconds per frame.
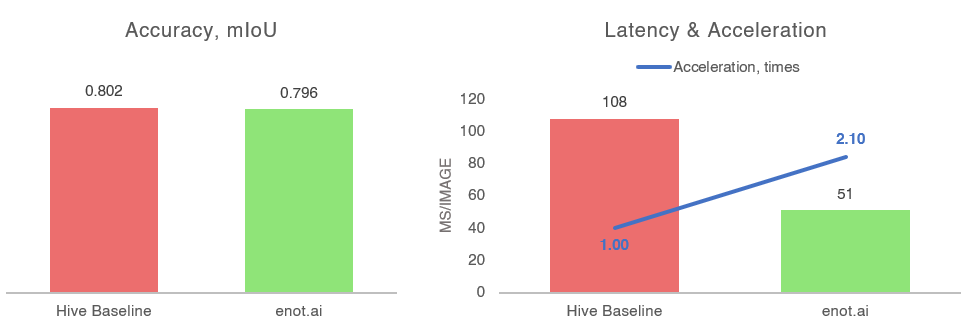
Summary:
After using enot.ai’s tools, Hive was able to accelerate their neural networks more than 2 times, thus enabling real-time processing. Since Yolo_v5s is already a very efficient neural network architecture, the results are quite significant.